There’s no shortage of ways for quantifying cyber risk, and that means there’s no shortage of people with strong opinions on which methods are superior. I see debates — battles, even — around this topic on LinkedIn at least once a week.
What I see less often is discussion around which parts of the risk management process each method is best suited for, and that’s a very important piece missing from these debates. Just like having the right tool for a particular job is crucial, there’s a right place in the process to use each quantification method. Sometimes, it even comes down to the specific type of risk you’re working with.
Just about every published risk management framework builds on this basic process:
- Identification
- Analysis
- Treatment definition and planning
- Treatment execution
- Re-evaluation
Different analysis methods are better suited at each phase, with the greatest concentration of quantification tools needed during the identification, analysis, and treatment phases. Let’s explore a few.
Quantifying cyber risk with ordinal scales
I see more disparaging remarks about quantifying risk with ordinal scales than any other quantification method. But used at the right point in your risk management framework, ordinal scales can be useful.
Here’s an example: Surveying all of the management teams in a large organization about all of the organization’s risks and which ones they think are the most critical is a great way to collect “directional information.” Ordinal scales are a good way to analyze and visualize the results. Is it perfect? No. But is it better than having no information at all and making a flat-out guess? Certainly.
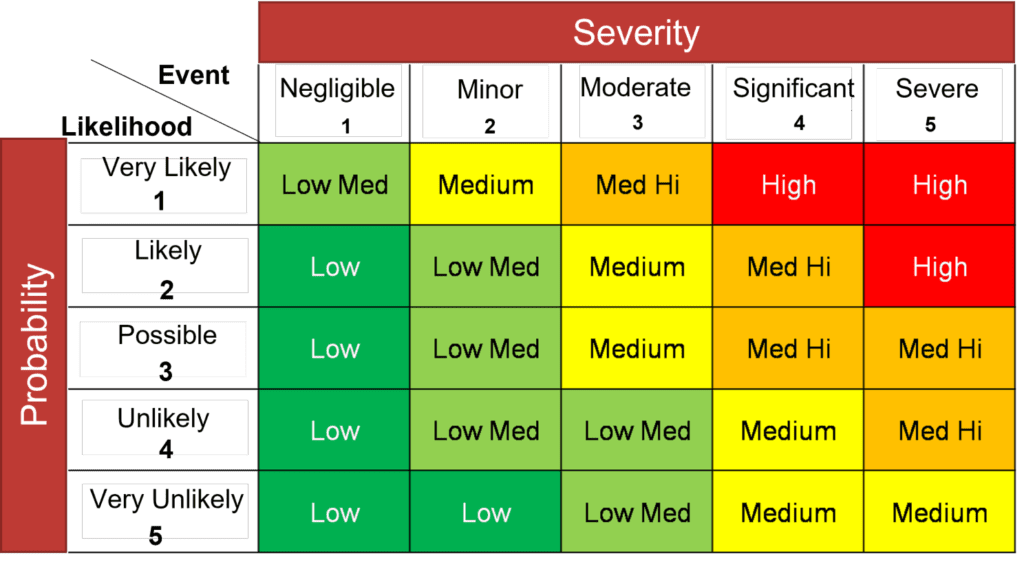
You’d never try to use an ordinal scale to make a multimillion dollar decision, but it is very useful for pointing you toward areas that warrant more investigation when you’re triaging hundreds or even thousands of risks. In the Treatment phase, many risks with low likelihood and low impact scores may be quickly moved to the “accepted risk” bucket while more stringent quantification effort is placed around entries that appear to pose a greater risk.
That said, even risks with low scores shouldn’t be ignored. Ransomware, for instance, was once viewed as a nuisance that affected a handful of system endpoints and would have received a low score at the time. Today, these types of attacks are among the most crippling threats an organization can face. Having a placeholder in your risk register for that risk allows it to be tracked during the Re-evaluation phase and as it changes over time.
Interval-based risk matrix quantification methods are better — and not much harder to build
Using interval-based risk matrices to quantify cyber risk are a step up from ordinal scales. This method takes a similar approach to ordinal scales, but goes a level deeper: adding probability and severity ranges.
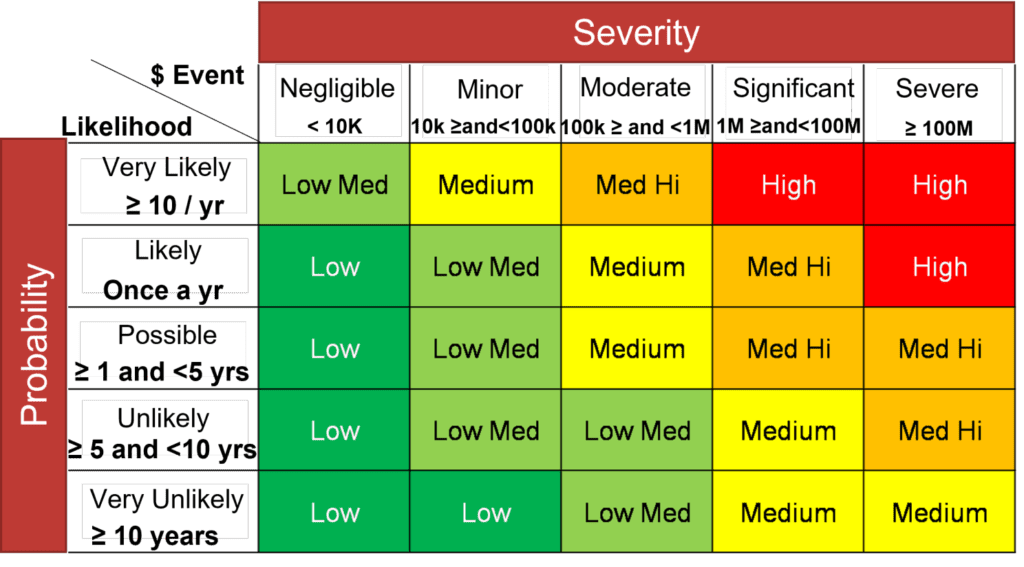
Ordinal scales are very limited in their use and provide very little insight. If you ask someone what their reasoning is you get 100 answers. Having these ranges provides a lot more information on what an actual loss may be in terms of dollars, which helps add much more context when analyzing and considering them. That leads to deeper discussions.
You still shouldn’t be grounding major strategic decisions in these results, but it’s a good way to illustrate the business impact of your risk.
And one thing to note: These scales need to vary by the business unit that they’re used to address. A $1 million USD loss in a single business unit may have a high impact, but the effects on the whole enterprise are probably negligible. If that same loss occurred across 100 business units all at once? Well, that’s a different story.
This is a good method to use in the Identification phase.
Monte Carlo simulations for quick triage
Let’s build a bit on the previous methods with something with stronger mathematical foundations: the Monte Carlo simulation.
Monte Carlo simulations only require slightly more data than ordinal scales and interval-based matrices and dramatically increases the accuracy of your risk estimates for your highest-impact risks.
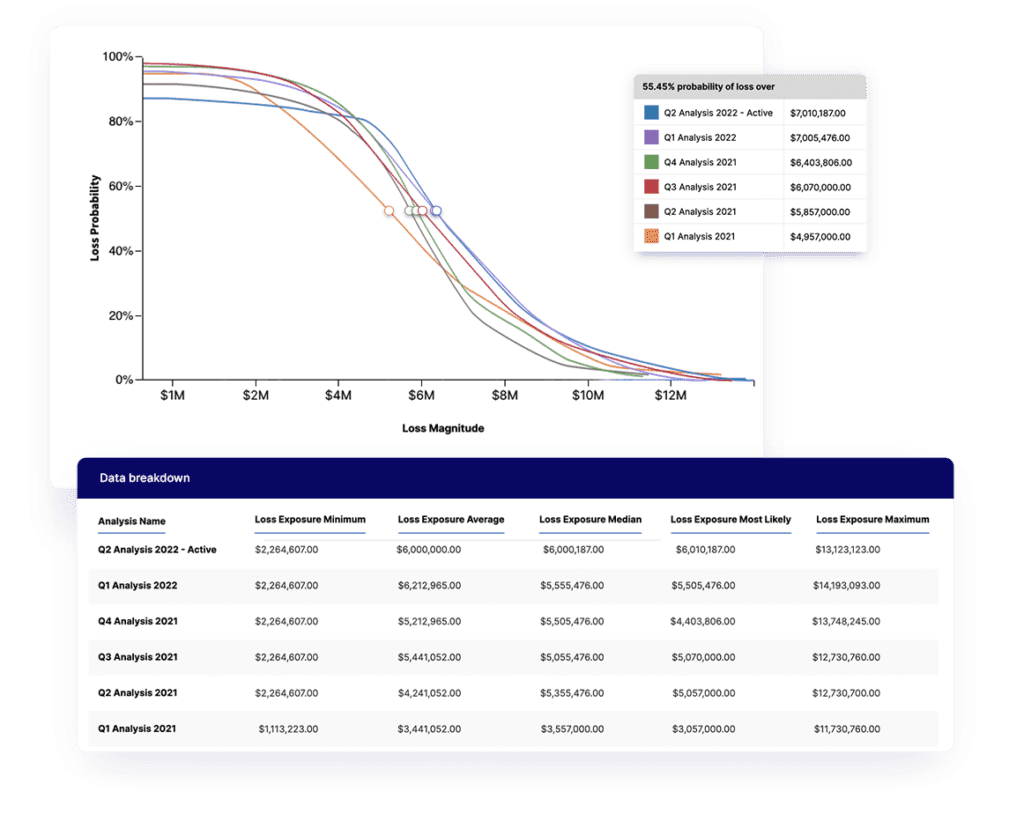
I first encountered Monte Carlo simulations in manufacturing and logistics simulations. In those situations, these models are used to plan factory and supply chain loads and throughput. This type of modeling improves the quality of estimations related to a simulation of possible outcomes, and it works just as well for risk scenarios as manufacturing and logistics.
These still probably won’t provide enough detail to make major decisions in the Treatment phase, but they’re a start to taking a much deeper dive into exploring risk when you get to that phase.
Quantification methods in the Treatment phase
The methods I have discussed so far are appropriate for the Identification and Assessment/Analysis phases. While they can also be useful in the Treatment phase, that phase typically requires more in-depth analysis as different treatments, trade-offs, and scenarios are considered.
Acceptance
If you’ve determined that a risk falls into a category where the risk of loss is so insignificant that it can simply be accepted, don’t waste your effort and resources on complex analysis. Just accept them and move on to more critical risks.
Avoidance
The Treatment phase needs a bit more examination than was done in the Identification and Assessment phases. Avoiding risks usually involves a trade-off of some sort — leaving a market, for instance, which leaves your organization unable to serve those customers, forces them to migrate to a competitor that is willing to take those risks, and impacts your bottom line.
So, while this decision-making process is not as difficult as examining transference or controls, it likely needs more scrutiny than the earlier phases
Transference
Here’s where a deeper analysis like Open FAIR for cyber risk comes into play.
In transference, risk analysts are looking to roll risk up at the enterprise level, along with any existing controls to secure lower risk and cyber risk insurance costs. The idea is that if you can effectively demonstrate to the insurer the true size of your risk exposure, you’ll be in a better position to negotiate for a lower premium.
I’ve seen more than one organization reduce their premium with a well-formed modeling and simulation of their risk, but there’s no way that’s going to happen if you’re presenting an ordinal scale of 1-5 rankings. This alone justifies the financial case for using quantitative methods like Open FAIR™.
Mitigation
Mitigation is the most complex phase for risk quantification. During mitigation, you’re evaluating the different controls and countermeasures you can implement for each risk you’re facing, as well as the various trade-offs in controls and countermeasures that need to be made.
With this type of quantification, the impact of mitigation techniques can be measured and in aggregate to derive the overall impact of both the risk and the efforts to address and manage it. It is also where using ordinal scales will result in some poorly informed and potentially risky decisions.
Treatment execution
Evaluating treatment execution usually relies on more traditional project management-type quantification. Here, we’re answering questions like “Did teams complete the work they were assigned?” and “Where did we end up with exceptions?”
Typically, a well-designed dashboard is sufficient to handle these questions, but you might also want to measure the impact of failed control implementations or the exposure resulting from granted exceptions.
The re-evaluation phase
This is the most exciting phase for us here at LogicGate. Governance, risk, and compliance programs are rich with data that can support evaluation of your previous assumptions and deliver real-time risk telemetry, enabling us to conduct continuous risk evaluation at the enterprise level. If a set of controls start to fail, the data will be fed into your models and give you a quick breakdown of how your risk posture has shifted.
There’s no silver bullet
So, the answer to which risk quantification method reigns supreme is … it depends. It depends on where you are in your risk management framework, and the attributes of the risk you’re facing itself. Sometimes, even organizational politics or long-established practices can determine which quantification methods are used.
The ultimate goal is to meet your organization where its capabilities are and help it best leverage the vast amount of data in their GRC and cyber risk quantification platforms, like LogicGate Risk Cloud®. With the right quantification capabilities in place, organizations can make the most informed risk decisions possible.
Click here to learn more about cyber risk quantification in Risk Cloud.